13:30-15:00, Wednesday, 21 February 2024, online, register here
The webinar, led by Dr Oliver Fisher and Professor Rachel Gomes from the University of Nottingham, features prominent academics discussing the application of AI and machine learning to improve bioremediation, resource recovery, and environmental protection. They showcased case studies from their respective work. Subsequently, a 30-minute panel session allowed attendees to engage with the panellists, asking questions related to the presentations and the overarching theme of the webinar. Speakers include:
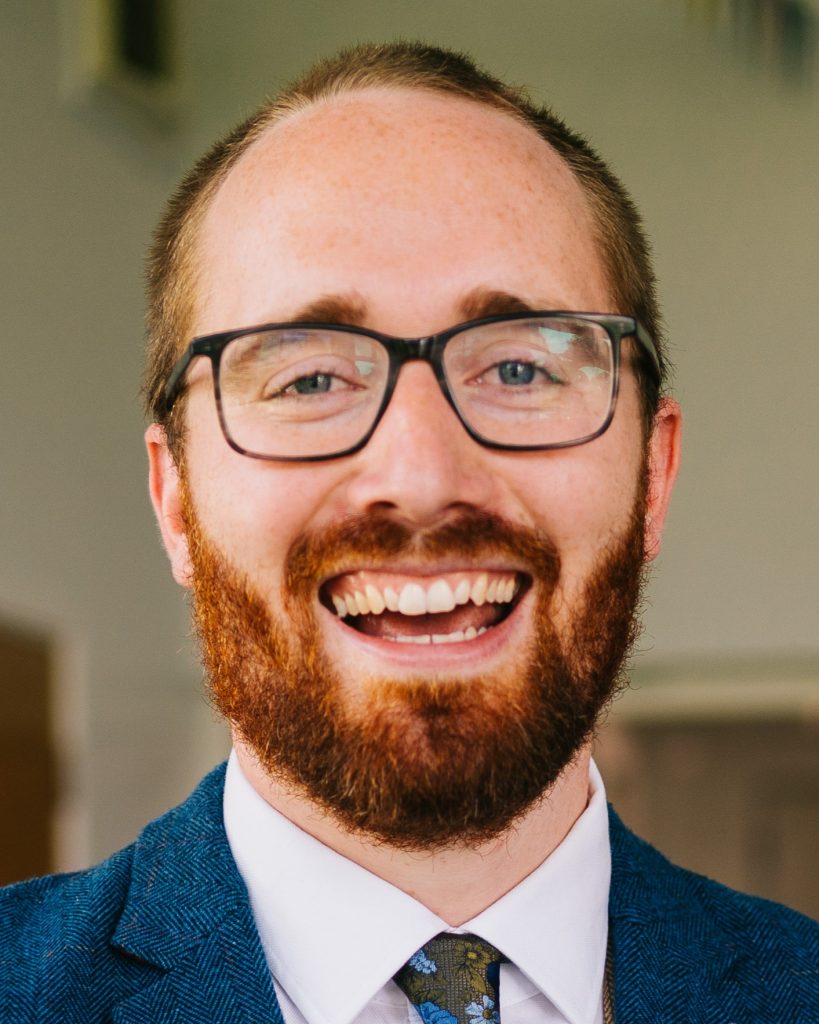
Dr Oliver Fisher is an Assistant Professor in Chemical and Environmental Engineering, specialising in leveraging digital technologies to advance a sustainable and inclusive circular economy. He holds an MEng (University of Nottingham, 2016) and a PhD (University of Nottingham, 2020) in Chemical Engineering. Between 2020-2022, Oliver worked as a Research Fellow and Knowledge Exchange Lead for Connected Everything, an EPSCR funded digital manufacturing network plus.From 2022-2023, Oliver was a Research Fellow at the University of Surrey working on ESPRC and Innovate UK funded projects. At Surrey, Oliver led the techno-economic analysis (TEA) and life cycle assessment (LCA) work package of the Flue2Chem Project, which aimed to develop a new UK supply chain to convert industrial waste gases into sustainable materials for consumer products. Separately, he also worked to develop new AI and machine learning tools to create a digital twin of a net zero biomanufacturing carbon capture and utilisation system..
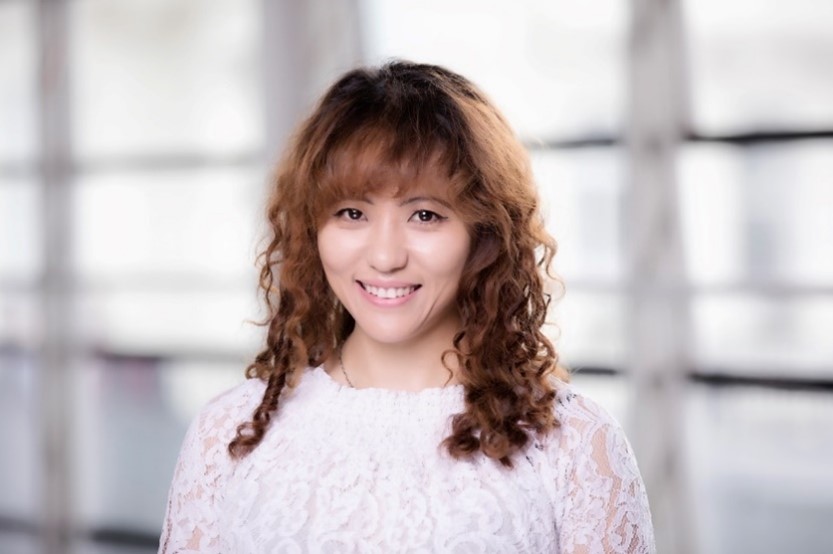
Dr Miao Guo is a Senior Lecturer (Associate Professor) in Department of Engineering at Kingâs College London. With a cross-disciplinary background in Life Sciences (PhD from Imperial College) and Chemical Engineering (postdoc/EPSRC Fellowship at Imperial College), her research interests sit at the interface of science and engineering, particularly in mathematical and computer algorithms, process systems engineering (PSE) methods and tools development, as well as novel PSE application areas e.g. waste-to-protein, biomanufacturing, digitalised toxicology. Collaborating with academic colleagues and industrial pioneers, her lab experimentally and computationally develops and optimises novel bioprocesses and waste-to-resource technologies.
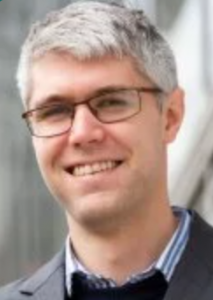
Dr Michael Short is a Senior Lecturer of Chemical and Process Systems Engineering at the University of Surrey in the School of Chemistry and Chemical Engineering and Sustainability Fellow in the Surrey Institute for Sustainability. His research expertise are in the development mathematical optimisation tools to create software for process systems for automated optimal sustainable, process design, renewable energy systems, policymaking, process integration, data analysis, and process control. His research team works on applying mathematical modelling and optimisation techniques to develop software for decision support in a wide range of fields including energy planning, bioenergy, renewable energy, water, heat integration, reactor design, real-time optimisation of industrial processes, and pharmaceuticals. He is lead investigator on an EPSRC project to apply AI and machine learning principles to anaerobic digestion: Artificial Intelligence Enabling Future Optimal Flexible Biogas Production for Net-Zero.
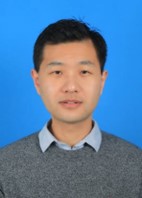
Dr Dongda Zhang is a Lecturer at the Department of Chemical Engineering, the University of Manchester. His research group focuses on the development of methodologies and practical applications for industrially focused mathematical modelling and data analytical techniques, aimed at understanding and operating complex chemical and biochemical processes. He is interested in exploring how advanced ‘digital technologies’, particularly integrated through interpretable machine learning and data intelligence techniques, along with rigorous mathematical analysis and physical modelling approaches, can accelerate novel process development and automate industrial process manufacturing. He has published over 60 research articles on chemical and biochemical process predictive modelling, optimisation, control, monitoring, and upscaling, and secured over ÂŁ1m from research councils and industry. He is an associate editor for Digital Chemical Engineering, a member of the Editorial Board for Biochemical Engineering Journal, a member of BBSRC Pool of Experts for Bioprocess Systems Engineering, and a member of the Industrial Management Board, Centre for Process Analytics and Control Technology.
Useful papers and resources:
Durkin A, Otte, L, Guo, M, 2024. Surrogate-based optimisation of process systems to recover resources from wastewater. Computers & Chemical Engineering. 182; 108584.
Considerations, challenges and opportunities for manufacturers when developing a data-driven model. By: Fisher OJ, Watson NJ, Escrig J, Witt R, Porcu L, Bacon D, Rigley M, Gomes RL, 2020. In: Computers and Chemical Engineering,140; 106881
Multiple target data-driven models to enable sustainable process manufacturing: An industrial bioprocess case study. By: Fisher OJ, Watson NJ, Porcu L, Bacon D, Rigley M, Gomes RL, 2021. In: Journal of Cleaner Production. 296; 126242
A data-driven modelling approach for process manufacturing systems: Data volume, variability, and visualisation for an industrial bioprocess. By: Fisher OJ, Watson NJ, Porcu L, Bacon D, Rigley M, Gomes RL, 2022. In: Biochemical Engineering Journal. 185; 208499
Investigating âgreynessâ of hybrid model for bioprocess predictive modelling. By: Alexander W. Rogers, Ziqi Song, Fernando Vega Ramon, Keju Jing, Dongda Zhang. In: Biochemical Engineering Journal. Volume 190, January 2023, 108761
A transfer learning approach for predictive modeling of bioprocesses using small data. By: Alexander W. Rogers, Fernando Vega-Ramon, Jiangtao Yan, Ehecatl A. del RĂo-Chanona, Keju Jing, Dongda Zhang. In: Biotechnology and Bioengineering. Volume119, Issue 2, Pages 411-422