Aim
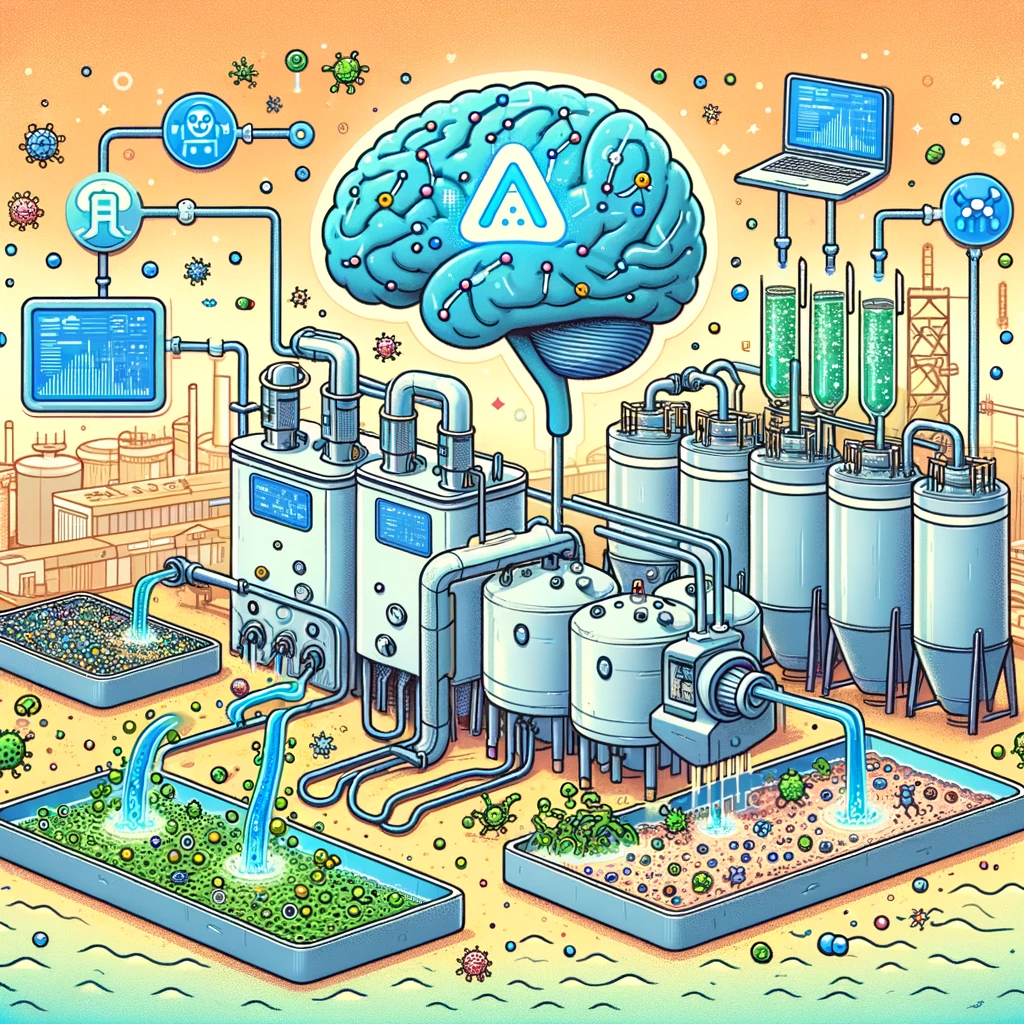
The bioeconomy continues to grow, offering an alternative to our fossil fuel-based economy and facilitating the transition to net zero, circular economy and/or industrial symbiosis. However, bioprocesses have complex dynamics and are subject to disturbances, which makes modelling bioprocesses challenging yet necessary for process understanding, model-based optimisation and scale-up. The biological nature of bioprocesses makes constructing models based on physical laws particularly challenging. The challenge is further compounded when utilising waste-based feedstocks for bioprocesses, which have variable composition and characteristics in time and space. Artificial intelligence (AI) and machine learning represent digital innovation and solutions for tackling emerging challenges in bioprocesses, such as resource specification and availability, parameter dimensionality, nonlinearity, risk mitigation, and complex metabolisms. Multivariate data analysis, deep learning, reinforcement learning, and other novel machine learning techniques start to complement and replace traditional data analysis approaches to accelerate bioprocess development and application.
This working group will accelerate trans-disciplinary collaboration, foster new collaborations between industry and academia and tackle emerging AI and machine learning modelling challenges that will underpin the creation of digitalised bioeconomy. As AI and machine learning is increasingly deployed within the bioeconomy, it is important to understand the social impact of its use in order to create an inclusive bioeconomy. This working group will also aim to understand the equality, diversity and inclusion considerations of using artificial intelligence and machine learning to progress bioprocesses development and application.
Interested?
Contact the working group co-ordinators, Dr Oliver Fisher oliver.fisher2[@]nottingham.ac.uk or Professor Rachel Gomes, rachel.gomes[@]nottingham.ac.uk.
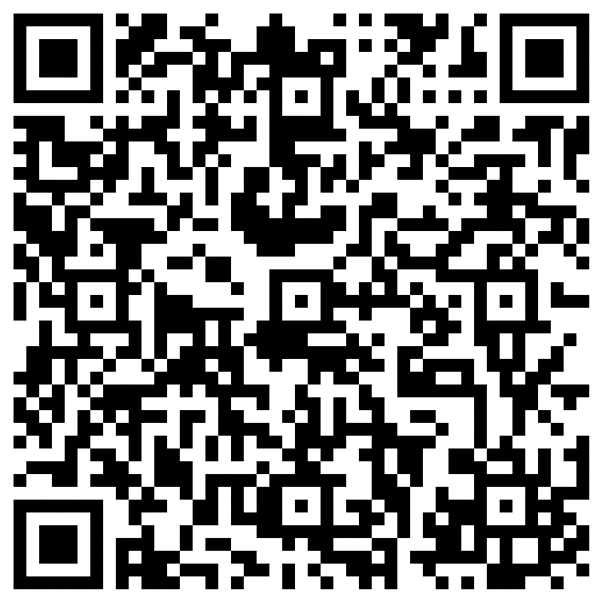
About
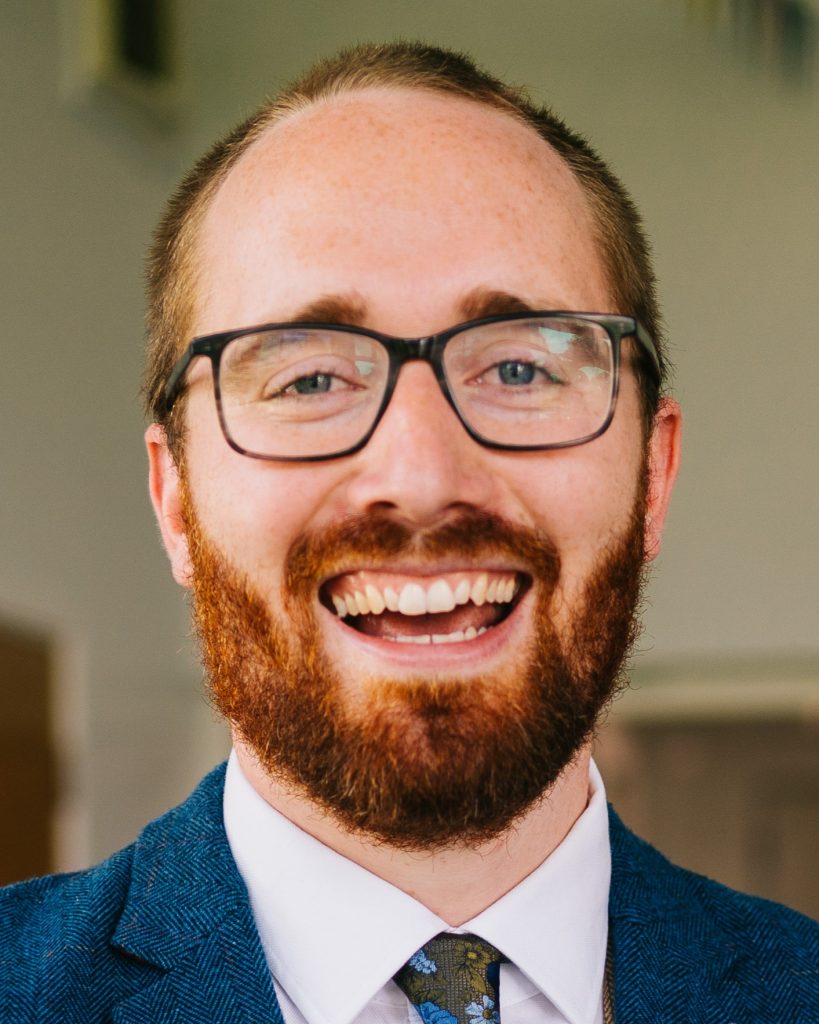
Oliver Fisher is an Assistant Professor in Chemical and Environmental Engineering, specialising in leveraging digital technologies to advance a sustainable and inclusive circular economy. He holds an MEng (University of Nottingham, 2016) and a PhD (University of Nottingham, 2020) in Chemical Engineering. Between 2020-2022, Oliver worked as a Research Fellow and Knowledge Exchange Lead for Connected Everything, an EPSRC-funded digital manufacturing network .From 2022-2023, Oliver was a Research Fellow at the University of Surrey working on ESPRC and Innovate UK funded projects. At Surrey, Oliver led the techno-economic analysis (TEA) and life cycle assessment (LCA) work package of the Flue2Chem Project, which aimed to develop a new UK supply chain to convert industrial waste gases into sustainable materials for consumer products. Separately, he also worked to develop new AI and machine learning tools to create a digital twin of a net zero biomanufacturing carbon capture and utilisation system.
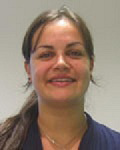
Rachel Gomes is a Professor in Chemical and Environmental Engineering researching intelligent resource use in process environments, and with a particular love for wastewater reuse, pollutant remediation and value recovery from process streams. In collaboration with multi-stakeholders and countries, particularly in the Global South, her research informs on future resource sustainability by understanding how water, energy and spent (waste) resources consumed by society can be more intelligently sourced and used, without depreciating process environments. Rachel is Head of the Food, Water, Waste Research Group and Lead for the University of Nottingham Interdisciplinary Research Cluster, Water Works comprising 140 academics. Rachel was named one of the Top 50 Women in Engineering on Sustainability by the Womenâs Engineering Society in 2020 and in the 2022 Birthday Honours List to mark the Queenâs Platinum Jubilee celebrations for her services to research and to education.
WG Activities
For WG Activities Report see HERE
Unlocking AI and Machine Learning’s Potential for Environmental Biotechnology 13:30-15:00, 21 February 2024. See HERE for more about the workshop and its outputs.
Get Ready for the AI Boost, by Angela Bywater, AD & Bioresources News, Issue 58, Winter 2024, extracted here.
Relevant Publications
Durkin A, Otte, L, Guo, M, 2024. Surrogate-based optimisation of process systems to recover resources from wastewater. Computers & Chemical Engineering. 182; 108584.
Fisher OJ, Watson NJ, Escrig J, Witt R, Porcu L, Bacon D, Rigley M, Gomes RL, 2020. Considerations, challenges and opportunities for manufacturers when developing a data-driven model. Computers & Chemical Engineering. 140; 106881
Fisher OJ, Watson NJ, Porcu L, Bacon D, Rigley M, Gomes RL, 2021. Multiple target data-driven models to enable sustainable process manufacturing: An industrial bioprocess case study. Journal of Cleaner Production. 296; 126242
Fisher OJ, Watson NJ, Porcu L, Bacon D, Rigley M, Gomes RL, 2022. A data-driven modelling approach for process manufacturing systems: Data volume, variability, and visualisation for an industrial bioprocess. Biochemical Engineering Journal. 185; 208499
GarduĆo-JimĂ©nez AL, DurĂĄn-Ălvarez JC, Gomes RL, 2022. Meta-analysis and machine learning to explore soil-water partitioning of common pharmaceuticals. Science of the Total Environment 155675.